Dancing with Our Algorithms
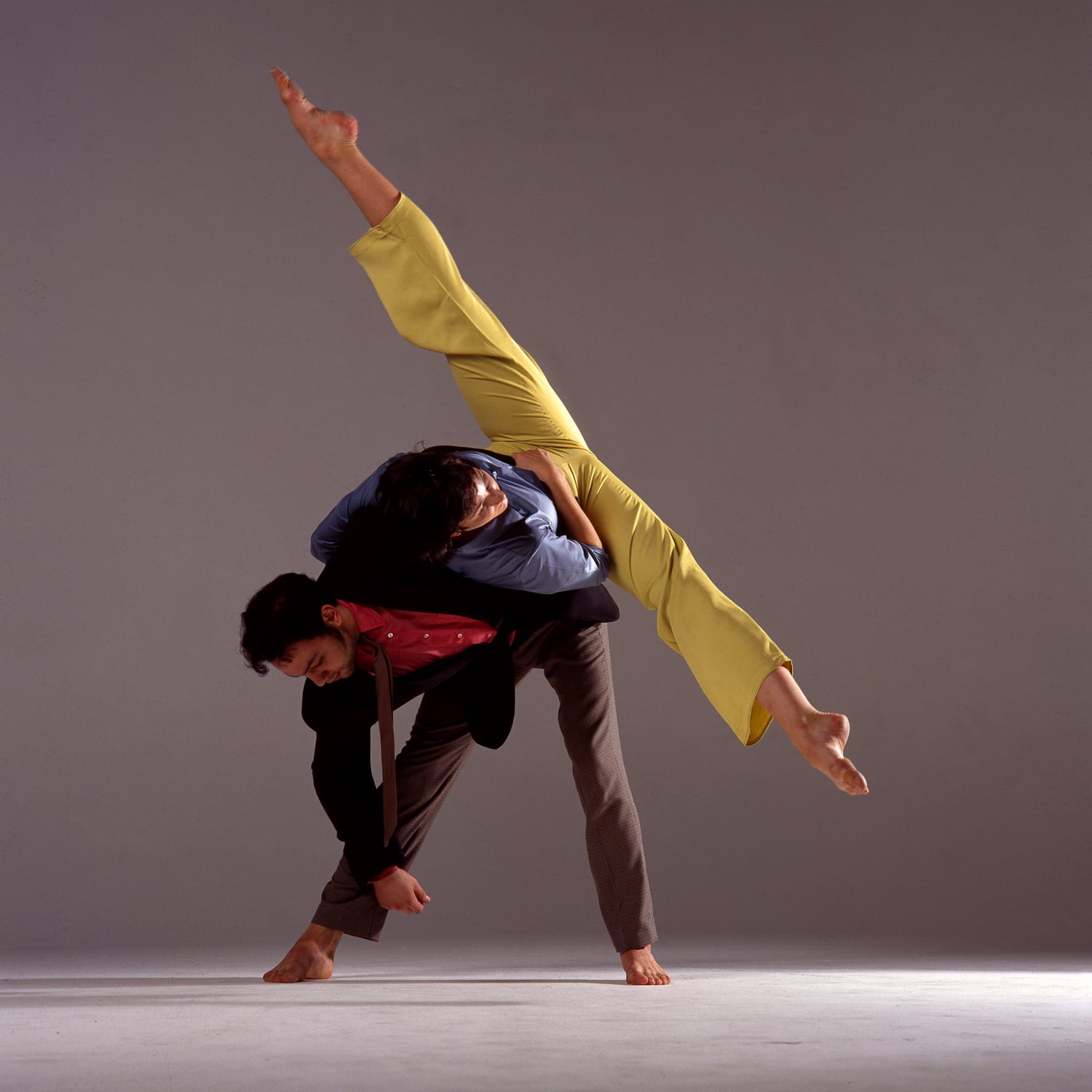
A couple of years ago we looked into algorithmic awareness and preferences when it comes to streaming content experiences, with sources including (but not limited to) Netflix, Youtube and Spotify. At the time we uncovered interesting insights into how people would like to interact with their recommendation engines, both actively and passively.
After further exploration during the ongoing rise of the algorithmically-sophisticated video gorilla known as Tik Tok, we’ve revisited our recommendations and found them still worthy.
Below are a few updated suggestions about how algorithms could become more transparent, more personal and ultimately more powerful, in ways that might seamlessly serve all those goals (and more):
Algorithms obviously operate on the basis of similarity - they recommend content which is by some degree similar to what we’ve already listened to or viewed. For a new user on a content provider’s site this isn’t necessarily a problem, as much of what they’re seeing feels new and different. However, more experienced users may find themselves looking at the same set of suggestions every time they log on. What if there were a way to either shrink or expand recommendation scope? Users should have the option to calibrate their algorithms either more narrowly (suggesting content very similar to their consumption history) or broadly (suggesting content only tangentially related to what they’ve watched before). We picture a sliding scale, that on one end would result in more familiar recommendations, and on the other end less familiar recommendations.
This additional flexibility might encourage habitually cautious users to engage with more diverse content — and satisfy more adventurous users who are constantly looking to broaden their horizons.
Our rec engines often know what content we will like before we do, yet we have no idea how they work. We are eager to understand more of the recommendation process — not necessarily the nitty gritty technical stuff, but the basic building blocks of what goes into each suggestion. Beyond any moral/ethical considerations (as those motivating the recent EU legislation), more transparency could also provide added value. For instance - access to an easy visualization that gives a window into why certain types of music were suggested, along with summaries of our unique listening histories, could enhance content experiences across the board.
Spotify has multiple metrics that define each song, from normal stats like tempo and length to more complex algorithmically-generated metrics like danceability or acousticness. This information drives recommendation using different statistics for different styles of playlists. Simply exposing these metrics for songs and playlists could add a new dimension to music enjoyment and searching.
We envision a drop-down option that would show which of our previous choices led the algorithm to make its suggestions. This kind of feature would help us understand the magic behind the recommendation process while boosting trust and satisfaction.
People want to be able to give algorithms more direct input on their recommendations. So our algorithms can, and should, elicit and respond to very specific feedback. Say you watch a horror movie one night with some friends, but you don’t actually like that genre. In most instances now, there’s no easy way to tell an algorithm to remove that behavior from your history and adjust the recommendations accordingly. Ideally users could avoid having to engage in a content navigation balancing act to “fix” their algorithms and bring them back to “normal”.
Features to tell websites you're not interested in content are becoming standard but what would be even better is to have preventive options that allow exploration and discovery without affecting the balance of the algorithm.
We think there’s a place for more categorized recommendations and for more flexibility within user profiles. The fundamental problem with one comprehensive algorithm is that our tastes vary, and there is currently no way of curating multiple algorithms. Some of us already actively interact with algorithms so that they’ll learn our tastes. Yet as tastes change (sometimes because of the algorithm) and are quite diverse, this results in excess need for interaction.
An improved interface might include the option to add sub-profiles to your Netflix or Spotify account — each with its own distinct tastes and “personality” — in which unique or semi-independent algorithms could operate. This would enhance the experience for people with highly variable content choice patterns.
What we’re proposing is simply a more collaborative way of thinking about content algorithms - as sometimes invisible allies, sometimes visible fellow travelers and ultimately partners in a dance where we alternate leading and following, willing to retain control or let go as the mood suits us. An optimistic yet realistic view that could benefit everyone - creators, distributors, subscribers, users - at least until the metaverse crashes the party.
Share this Post
Build new levels of engagement and connection with your viewers - get in touch to find out more about Lumiere and our insights solutions.